belum terdaftar anggota QQEMAS?
JOIN NOWLatest Posts
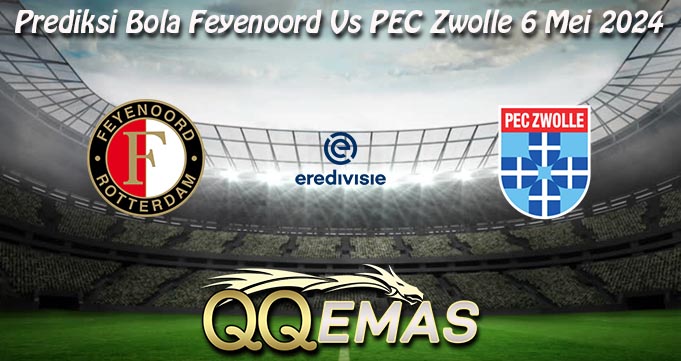
Prediksi Bola Feyenoord Vs PEC Zwolle 6 Mei 2024
Prediksi Bola Feyenoord Vs PEC Zwolle 6 Mei 2024 – Pada lanjutan kompetisi Eredivisie pekan ini hadir matchday 32. Berlangsung De Kuip Stadium Feyenoord selaku... Read More »
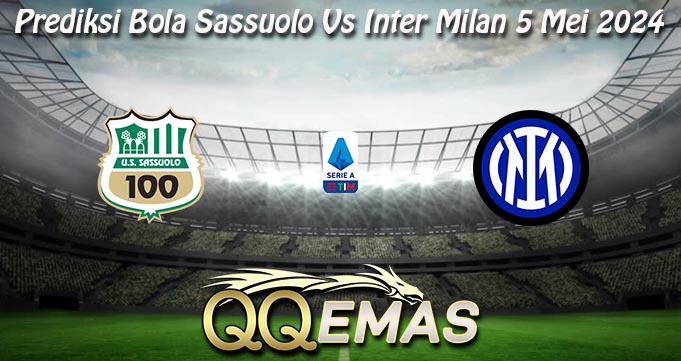
Prediksi Bola Sassuolo Vs Inter Milan 5 Mei 2024
Prediksi Bola Sassuolo Vs Inter Milan 5 Mei 2024 – Stadio Citta del Tricolore menhadirkan laga kompetisi Serie A giornata 35. Sassuolo yang selaku tuan... Read More »
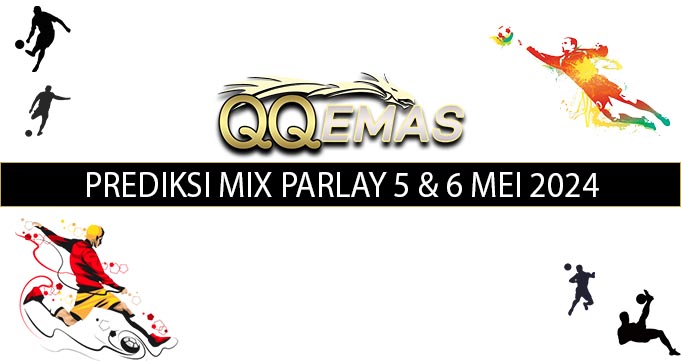
Bocoran Mix Parlay 5 Dan 6 Mei 2024
Situs bola Terpercaya QQ Emas akan memberikan Bocoran Mix Parlay 5 Dan 6 Mei 2024 untuk para member setia situs kami. Dalam rangkuman ini kami... Read More »
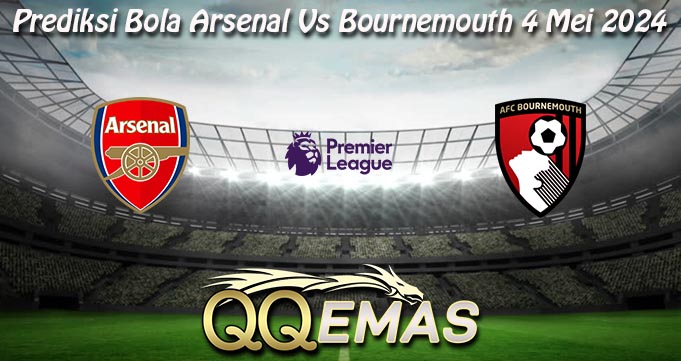
Prediksi Bola Arsenal Vs Bournemouth 4 Mei 2024
Prediksi Bola Arsenal Vs Bournemouth 4 Mei 2024 – Kompetisi Premier League akhir pekan ini memasuki matchday 36. Berlangsung Emirates Stadium Arsenal selaku tuan rumah... Read More »
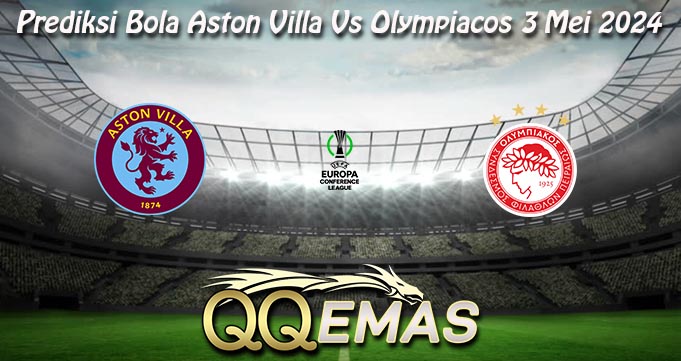
Prediksi Bola Aston Villa Vs Olympiacos 3 Mei 2024
Prediksi Bola Aston Villa Vs Olympiacos 3 Mei 2024 – Villa Park pekan ini mengelar laga kompetisi Europa Conference League. Leg pertama babak semifinal Aston... Read More »
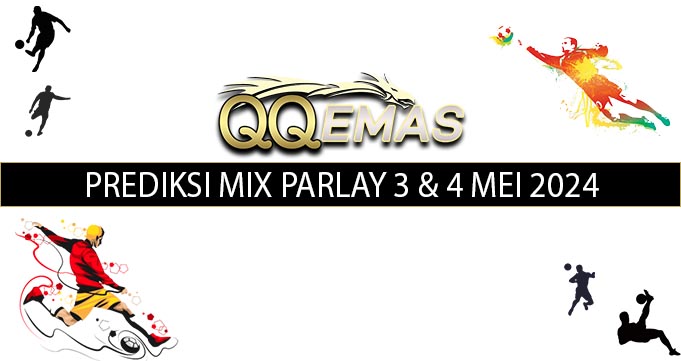
Bocoran Mix Parlay 3 Dan 4 Mei 2024
Situs bola Terpercaya QQ Emas akan memberikan Bocoran Mix Parlay 3 Dan 4 Mei 2024 untuk para member setia situs kami. Dalam rangkuman ini kami... Read More »
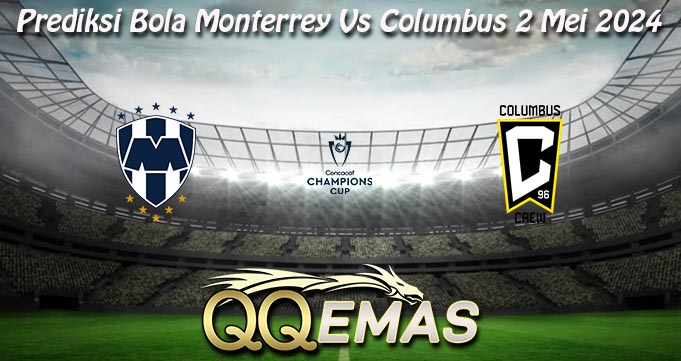
Prediksi Bola Monterrey Vs Columbus 2 Mei 2024
Prediksi Bola Monterrey Vs Columbus 2 Mei 2024 – Estadio BBVA mengelar pertandingan seri babak semifinal CONCACAF Champions Cup. Leg kedua ini Monterrey giliran turun... Read More »
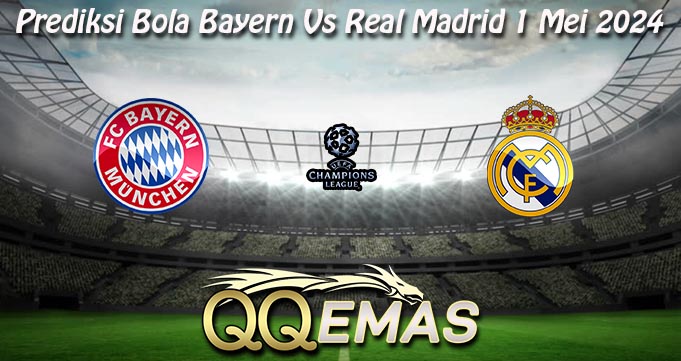
Prediksi Bola Bayern Vs Real Madrid 1 Mei 2024
Prediksi Bola Bayern Vs Real Madrid 1 Mei 2024 – Kompetisi Liga Champions tengah pekan ini memasuki babak semifinal. Leg pertama berlangsung Allianz Arena Bayern... Read More »
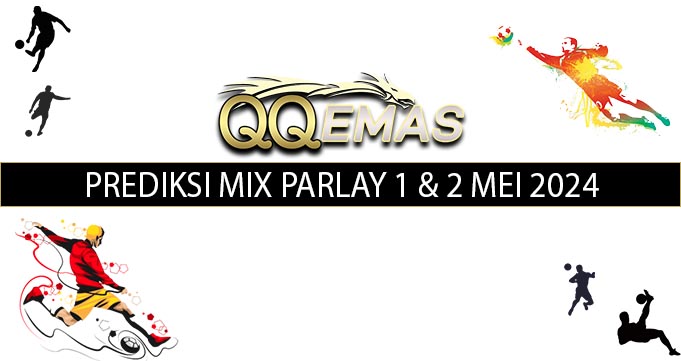
Bocoran Mix Parlay 1 Dan 2 Mei 2024
Situs bola Terpercaya QQ Emas akan memberikan Bocoran Mix Parlay 1 Dan 2 Mei 2024 untuk para member setia situs kami. Dalam rangkuman ini kami... Read More »
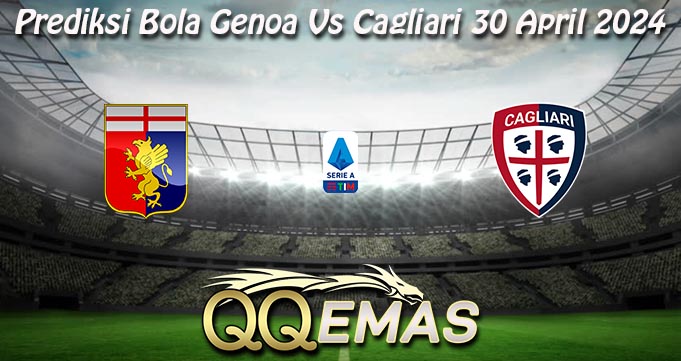
Prediksi Bola Genoa Vs Cagliari 30 April 2024
Prediksi Bola Genoa Vs Cagliari 30 April 2024 – Persaingan sengit juga terjadi pada papan bawah kompetisi Serie A. Berlangsung Luigi Ferraris Stadium Genoa selaku... Read More »